Can we predict Traffic Flow Using Neural Network?
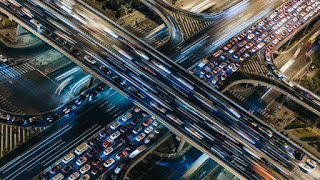
Ongoing traffic volume expectation assumes a fundamental job in proactive system the executives, and many estimating models have been proposed to address this issue in the writing. Notwithstanding, the greater part of them experience the ill effects of the powerlessness to completely utilize the rich data in rush hour gridlock information to produce proficient and precise traffic expectations for a more drawn out term (i.e., 7-day forecasts at a 5-min interim). We center around anticipating multi-step continuous traffic volume utilizing two kinds of Long Short-Term Memory (LSTM) systems: many-to-one LSTM and many-to-numerous LSTM by making an adaptable group guaging framework that consolidates quantities of neural system and forecasts out of interjection. Considering the enormous measure of information focuses in the current dataset, so as to address one of the run of the mill concerns we have with RNN models regarding longer preparing time, we do examining on the first datas...